목적
패션 데이터셋을 활용하여 가방, 셔츠, 스커트 등을 분류합니다.
환경설정
- 데이터 파이프 라인 : 데이터 로드시 과부하를 완화하기 위하여 데이터 파이프라인을 구축합니다.
- 조기종료 설정 : 학습이 개선되지 않으면 조기종료 콜백을 설정하여 중지합니다.
- TensorBoard 모니터링 : TensorBoard와 연결하여 학습 진행 상태를 모니터링 할 수 있도록 합니다.
데이터셋
아래와 같이 dataset 디렉토리 안에 가방, 셔츠, 스커트의 폴더별로 나누어져있으며 이미지 파일이 있습니다.
├─dataset
│ ├─bag
│ ├─shirt
│ └─skirt
└─runs
└─fashion_classification
데이터 로드
import os
import time
from PIL import Image
import numpy as np
import matplotlib.pyplot as plt
import random
import torch
import torch.nn as nn
from torch import optim, cuda
from torch.utils.data import DataLoader
from torch.utils.tensorboard import SummaryWriter
from torchvision import datasets, models, transforms
from torchvision.utils import make_grid
import matplotlib.pyplot as plt
from torchsummary import summary
BASE_DIR = os.getcwd()
DATASET_PATH = os.path.join(BASE_DIR, 'dataset')
MODE_PATH = os.path.join(BASE_DIR, 'models')
npy 파일을 체크해주는 이유는 첫번째 훈련시 이미지 경로에서 npy 파일을 만들고 그 다음 훈련때부터는 npy 파일만 로드하여 시간을 절약하기 위함입니다.
classes = []
dataset = []
for fullpath, dirnames, filenames in os.walk(DATASET_PATH):
if DATASET_PATH == fullpath:
classes = dirnames
continue
label_name = fullpath.split(os.path.sep)[-1]
for filename in filenames:
if filename.find('.npy') != -1:
continue
class_index = classes.index(label_name)
dataset.append((os.path.join(fullpath, filename), class_index))
random.shuffle(dataset)
total_len = len(dataset)
train_ratio = int(total_len * 0.8)
train_data = dataset[:train_ratio]
test_data = dataset[train_ratio:]
데이터 파이프 라인
전부 데이터를 가져와서 메모리에 갖고 있기에는 부하가 있기때문에 훈련시 배치 사이즈만큼 가져와서 가공할 수 있도록 데이터 파이프 라인을 만들어 보기로 합니다. 위에서 언급했듯이 첫번째 훈련시 이미지 경로에서 npy 파일을 만들고 그 다음 훈련때부터는 npy 파일만 로드하여 시간을 데이터 로드 시간을 절약하도록 합니다.
class Dataset(object):
def __init__(self, transforms, dataset):
self.transforms = transforms
self.dataset = dataset
def __getitem__(self, idx):
img_path, label = self.dataset[idx]
fullpath, extension = os.path.splitext(img_path)
npy_path = fullpath + '.npy'
if os.path.exists(npy_path):
np_data = np.load(npy_path)
img = Image.fromarray(np.uint8(np_data))
else:
img = Image.open(img_path).convert("RGB")
np_data = np.asarray(img)
np.save(npy_path, np_data)
if self.transforms is not None:
img = self.transforms(img)
return img, label
def __len__(self):
return len(self.dataset)
transform = transforms.Compose(
[
transforms.Resize((224, 224)),
transforms.ToTensor(),
transforms.Normalize((0.5, 0.5, 0.5), (0.5, 0.5, 0.5))
]
)
훈련 및 평가 데이터 파이프라인을 생성합니다.
train_dataset = Dataset(transform, train_data)
test_dataset = Dataset(transform, test_data)
train_loader = torch.utils.data.DataLoader(train_dataset, batch_size=20)
test_loader = torch.utils.data.DataLoader(test_dataset, batch_size=5)
데이터 시각화
이미지 한개만 시각화를 해보도록합니다.
dataloader = iter(train_loader)
imgs, target = dataloader.next()
tf = transforms.ToPILImage()
pil_img = tf(imgs[0])
plt.figure()
plt.imshow(pil_img)
plt.colorbar()
plt.gca().grid(False)
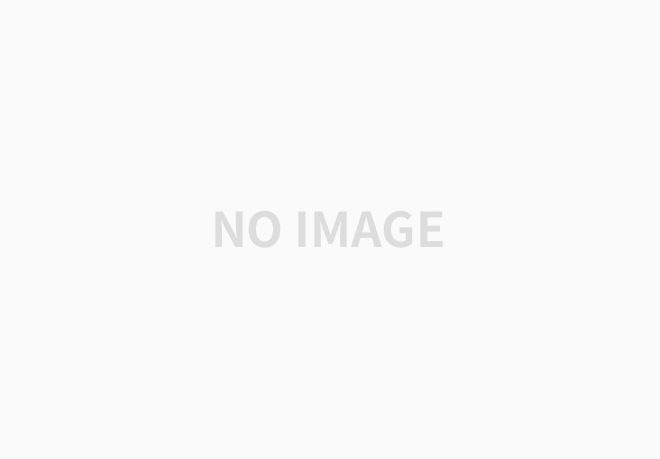
모델 정의
learning_rate = 0.001
device = torch.device('cuda') if torch.cuda.is_available() else torch.device('cpu')
device
vgg19 모델을 참고하였다.
class Net(nn.Module):
def __init__(self):
super(Net, self).__init__()
self.conv = nn.Sequential(
nn.Conv2d(3, 64, 3, padding=1),nn.LeakyReLU(0.2),
nn.Conv2d(64, 64, 3, padding=1),nn.LeakyReLU(0.2),
nn.MaxPool2d(2, 2),
nn.Conv2d(64, 128, 3, padding=1),nn.LeakyReLU(0.2),
nn.Conv2d(128, 128, 3, padding=1),nn.LeakyReLU(0.2),
nn.MaxPool2d(2, 2),
nn.Conv2d(128, 256, 3, padding=1),nn.LeakyReLU(0.2),
nn.Conv2d(256, 256, 3, padding=1),nn.LeakyReLU(0.2),
nn.Conv2d(256, 256, 3, padding=1),nn.LeakyReLU(0.2),
nn.MaxPool2d(2, 2),
nn.Conv2d(256, 512, 3, padding=1),nn.LeakyReLU(0.2),
nn.Conv2d(512, 512, 3, padding=1),nn.LeakyReLU(0.2),
nn.Conv2d(512, 512, 3, padding=1),nn.LeakyReLU(0.2),
nn.MaxPool2d(2, 2),
nn.Conv2d(512, 512, 3, padding=1),nn.LeakyReLU(0.2),
nn.Conv2d(512, 512, 3, padding=1),nn.LeakyReLU(0.2),
nn.Conv2d(512, 512, 3, padding=1),nn.LeakyReLU(0.2),
nn.MaxPool2d(2, 2)
)
self.avg_pool = nn.AvgPool2d(7)
self.classifier = nn.Linear(512, 10)
def forward(self, x):
features = self.conv(x)
x = self.avg_pool(features)
x = x.view(features.size(0), -1)
x = self.classifier(x)
return x, features
model = Net().to(device)
model
Net(
(conv): Sequential(
(0): Conv2d(3, 64, kernel_size=(3, 3), stride=(1, 1), padding=(1, 1))
(1): LeakyReLU(negative_slope=0.2)
(2): Conv2d(64, 64, kernel_size=(3, 3), stride=(1, 1), padding=(1, 1))
(3): LeakyReLU(negative_slope=0.2)
(4): MaxPool2d(kernel_size=2, stride=2, padding=0, dilation=1, ceil_mode=False)
(5): Conv2d(64, 128, kernel_size=(3, 3), stride=(1, 1), padding=(1, 1))
(6): LeakyReLU(negative_slope=0.2)
(7): Conv2d(128, 128, kernel_size=(3, 3), stride=(1, 1), padding=(1, 1))
(8): LeakyReLU(negative_slope=0.2)
(9): MaxPool2d(kernel_size=2, stride=2, padding=0, dilation=1, ceil_mode=False)
(10): Conv2d(128, 256, kernel_size=(3, 3), stride=(1, 1), padding=(1, 1))
(11): LeakyReLU(negative_slope=0.2)
(12): Conv2d(256, 256, kernel_size=(3, 3), stride=(1, 1), padding=(1, 1))
(13): LeakyReLU(negative_slope=0.2)
(14): Conv2d(256, 256, kernel_size=(3, 3), stride=(1, 1), padding=(1, 1))
(15): LeakyReLU(negative_slope=0.2)
(16): MaxPool2d(kernel_size=2, stride=2, padding=0, dilation=1, ceil_mode=False)
(17): Conv2d(256, 512, kernel_size=(3, 3), stride=(1, 1), padding=(1, 1))
(18): LeakyReLU(negative_slope=0.2)
(19): Conv2d(512, 512, kernel_size=(3, 3), stride=(1, 1), padding=(1, 1))
(20): LeakyReLU(negative_slope=0.2)
(21): Conv2d(512, 512, kernel_size=(3, 3), stride=(1, 1), padding=(1, 1))
(22): LeakyReLU(negative_slope=0.2)
(23): MaxPool2d(kernel_size=2, stride=2, padding=0, dilation=1, ceil_mode=False)
(24): Conv2d(512, 512, kernel_size=(3, 3), stride=(1, 1), padding=(1, 1))
(25): LeakyReLU(negative_slope=0.2)
(26): Conv2d(512, 512, kernel_size=(3, 3), stride=(1, 1), padding=(1, 1))
(27): LeakyReLU(negative_slope=0.2)
(28): Conv2d(512, 512, kernel_size=(3, 3), stride=(1, 1), padding=(1, 1))
(29): LeakyReLU(negative_slope=0.2)
(30): MaxPool2d(kernel_size=2, stride=2, padding=0, dilation=1, ceil_mode=False)
)
(avg_pool): AvgPool2d(kernel_size=7, stride=7, padding=0)
(classifier): Linear(in_features=512, out_features=10, bias=True)
)
criterion = torch.nn.CrossEntropyLoss().to(device)
optimizer = torch.optim.Adam(model.parameters(), lr=learning_rate)
summary(model.to(device), (3, 224, 224))
----------------------------------------------------------------
Layer (type) Output Shape Param #
================================================================
Conv2d-1 [-1, 64, 224, 224] 1,792
LeakyReLU-2 [-1, 64, 224, 224] 0
Conv2d-3 [-1, 64, 224, 224] 36,928
LeakyReLU-4 [-1, 64, 224, 224] 0
MaxPool2d-5 [-1, 64, 112, 112] 0
Conv2d-6 [-1, 128, 112, 112] 73,856
LeakyReLU-7 [-1, 128, 112, 112] 0
Conv2d-8 [-1, 128, 112, 112] 147,584
LeakyReLU-9 [-1, 128, 112, 112] 0
MaxPool2d-10 [-1, 128, 56, 56] 0
Conv2d-11 [-1, 256, 56, 56] 295,168
LeakyReLU-12 [-1, 256, 56, 56] 0
Conv2d-13 [-1, 256, 56, 56] 590,080
LeakyReLU-14 [-1, 256, 56, 56] 0
Conv2d-15 [-1, 256, 56, 56] 590,080
LeakyReLU-16 [-1, 256, 56, 56] 0
MaxPool2d-17 [-1, 256, 28, 28] 0
Conv2d-18 [-1, 512, 28, 28] 1,180,160
LeakyReLU-19 [-1, 512, 28, 28] 0
Conv2d-20 [-1, 512, 28, 28] 2,359,808
LeakyReLU-21 [-1, 512, 28, 28] 0
Conv2d-22 [-1, 512, 28, 28] 2,359,808
LeakyReLU-23 [-1, 512, 28, 28] 0
MaxPool2d-24 [-1, 512, 14, 14] 0
Conv2d-25 [-1, 512, 14, 14] 2,359,808
LeakyReLU-26 [-1, 512, 14, 14] 0
Conv2d-27 [-1, 512, 14, 14] 2,359,808
LeakyReLU-28 [-1, 512, 14, 14] 0
Conv2d-29 [-1, 512, 14, 14] 2,359,808
LeakyReLU-30 [-1, 512, 14, 14] 0
MaxPool2d-31 [-1, 512, 7, 7] 0
AvgPool2d-32 [-1, 512, 1, 1] 0
Linear-33 [-1, 10] 5,130
================================================================
Total params: 14,719,818
Trainable params: 14,719,818
Non-trainable params: 0
----------------------------------------------------------------
Input size (MB): 0.57
Forward/backward pass size (MB): 218.40
Params size (MB): 56.15
Estimated Total Size (MB): 275.12
----------------------------------------------------------------
조기종료 콜백 설정
class EarlyStopping:
"""Early stops the training if validation loss doesn't improve after a given patience."""
def __init__(self, patience=10, verbose=False, delta=0, path='checkpoint.pt', trace_func=print):
"""
Args:
patience (int): How long to wait after last time validation loss improved.
Default: 7
verbose (bool): If True, prints a message for each validation loss improvement.
Default: False
delta (float): Minimum change in the monitored quantity to qualify as an improvement.
Default: 0
path (str): Path for the checkpoint to be saved to.
Default: 'checkpoint.pt'
trace_func (function): trace print function.
Default: print
"""
self.patience = patience
self.verbose = verbose
self.counter = 0
self.best_score = None
self.early_stop = False
self.val_loss_min = np.Inf
self.delta = delta
self.path = path
self.trace_func = trace_func
def __call__(self, val_loss, model):
score = -val_loss
if self.best_score is None:
self.best_score = score
self.save_checkpoint(val_loss, model)
elif score < self.best_score + self.delta:
self.counter += 1
self.trace_func(f'EarlyStopping counter: {self.counter} out of {self.patience}')
if self.counter >= self.patience:
self.early_stop = True
else:
self.best_score = score
self.save_checkpoint(val_loss, model)
self.counter = 0
def save_checkpoint(self, val_loss, model):
'''Saves model when validation loss decrease.'''
if self.verbose:
self.trace_func(f'Validation loss decreased ({self.val_loss_min:.6f} --> {val_loss:.6f}). Saving model ...')
torch.save(model.state_dict(), self.path)
self.val_loss_min = val_loss
TensorBoard 처리
writer = SummaryWriter('runs/fashion_classification')
def matplotlib_imshow(img, one_channel=False):
if one_channel:
img = img.mean(dim=0)
img = img / 2 + 0.5
npimg = img.cpu().numpy()
if one_channel:
plt.imshow(npimg, cmap="Greys")
else:
plt.imshow(np.transpose(npimg, (1, 2, 0)))
def images_to_probs(model, images):
output,f = model(images)
_, preds_tensor = torch.max(output, 1)
preds = np.squeeze(preds_tensor.cpu().numpy())
return preds, [nn.functional.softmax(el, dim=0)[i].item() for i, el in zip(preds, output)]
def plot_classes_preds(model, images, labels):
preds, probs = images_to_probs(model, images)
fig = plt.figure(figsize=(12, 48))
for idx in np.arange(4):
if len(images) - 1 < idx:
break
ax = fig.add_subplot(1, 4, idx+1, xticks=[], yticks=[])
matplotlib_imshow(images[idx], one_channel=True)
ax.set_title("{0}, {1:.1f}%\n(label: {2})".format(
classes[preds[idx]],
probs[idx] * 100.0,
classes[labels[idx]]), color=("green" if preds[idx]==labels[idx].item() else "red"))
return fig
학습하기
훈련을 시작합니다.
def train_model(model, n_epochs):
train_losses = []
valid_losses = []
early_stopping = EarlyStopping(verbose = True)
for epoch in range(n_epochs):
epoch_loss = 0
start = time.time()
model.train()
for batch, (data, target) in enumerate(train_loader, 1):
data = data.to(device)
target = target.to(device)
output, f = model(data)
loss = criterion(output, target)
optimizer.zero_grad()
loss.backward()
optimizer.step()
epoch_loss += loss
if batch % 100 == 0:
writer.add_scalar('training loss', epoch_loss / 100, epoch * len(train_loader) + batch)
writer.add_figure('predictions vs. actuals', plot_classes_preds(model, data, target), global_step=epoch * len(train_loader) + batch)
print(f'epoch : {epoch+1}, Loss : {epoch_loss}, time : {time.time() - start}')
model.eval()
for data, target in test_loader :
data = data.to(device)
target = target.to(device)
output, f = model(data)
loss = criterion(output, target)
valid_losses.append(loss.item())
train_loss = np.average(train_losses)
valid_loss = np.average(valid_losses)
epoch_len = len(str(n_epochs))
train_losses = []
valid_losses = []
early_stopping(valid_loss, model)
if early_stopping.early_stop:
print("Early stopping")
break
n_epochs = 50
train_model(model, n_epochs)
epoch : 2, Loss : 211.1282958984375, time : 91.98287105560303
Validation loss decreased (inf --> 0.787457). Saving model ...
epoch : 3, Loss : 195.0592041015625, time : 77.08459329605103
Validation loss decreased (0.787457 --> 0.767096). Saving model ...
epoch : 4, Loss : 169.17291259765625, time : 76.82666802406311
Validation loss decreased (0.767096 --> 0.688257). Saving model ...
epoch : 5, Loss : 118.1400146484375, time : 76.96125864982605
...<생략>
학습 모니터링
tensorboard --logdir runs
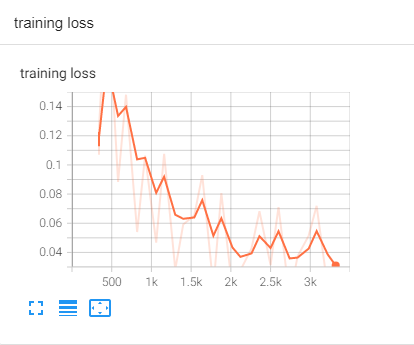
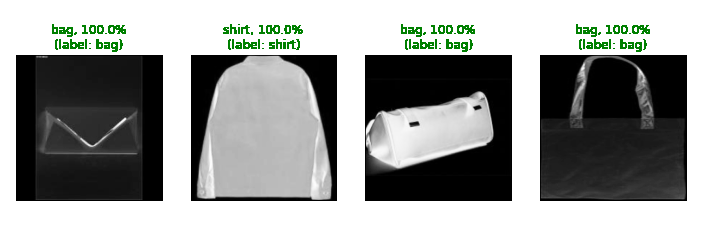
평가하기
columns = 5
rows = 3
fig = plt.figure(figsize=(20,10))
model.eval()
for i in range(1, columns*rows+1):
idx = np.random.randint(len(test_data))
img_path, class_index = test_data[idx]
pil_img = Image.open(img_path).convert('RGB')
img = transform(pil_img).unsqueeze(dim=0).to(device)
output,f = model(img)
_, argmax = torch.max(output, 1)
pred = classes[argmax.item()]
label = classes[class_index]
fig.add_subplot(rows, columns, i)
plt.title(pred)
plt.imshow(pil_img)
plt.axis('off')
plt.show()
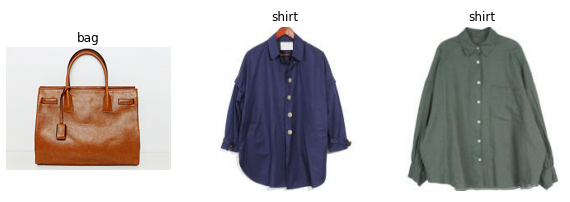
'AI 인공지능 > AI Vision' 카테고리의 다른 글
YOLOv7를 활용한 Object Detection (0) | 2022.11.21 |
---|---|
이미지 유사도 embedding (1) | 2021.12.29 |
Fashion MNIST (0) | 2021.09.12 |
Object Detection 개념 (0) | 2021.09.12 |
Faster-RCNN (0) | 2021.09.09 |